The Art and Science of Data Analysis: Unlocking Insights for a Data-Driven World
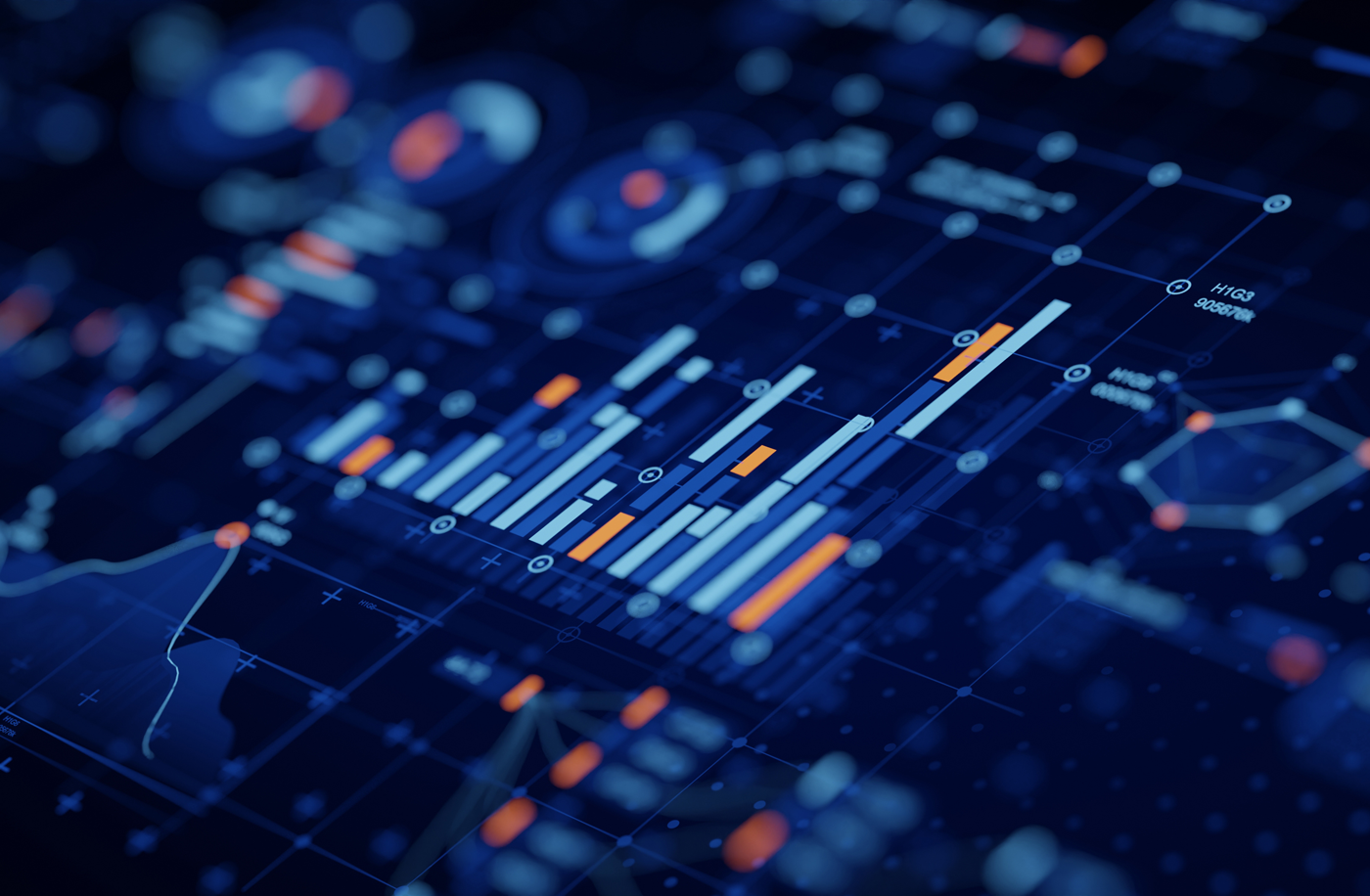
Data analysis has emerged as one of the most critical skills in the modern era, shaping industries, driving innovations, and enabling organizations to make informed decisions. As businesses generate an unprecedented amount of data daily, the ability to analyze and derive meaningful insights has become indispensable. This article explores the essence of data analysis, its methodologies, tools, and real-world applications while emphasizing its significance in today’s rapidly evolving landscape.
What Is Data Analysis?
Data analysis refers to the process of inspecting, cleaning, transforming, and modeling data to uncover useful information, draw conclusions, and support decision-making. It lies at the intersection of art and science, requiring both creativity and technical proficiency. Data analysts interpret raw data, identify patterns, and present findings in ways that stakeholders can understand and act upon.
The Stages of Data Analysis
- Data Collection
The foundation of data analysis is collecting accurate and relevant data. This may involve surveys, sensors, web scraping, or databases. Without high-quality data, the results of the analysis may be flawed. - Data Cleaning
Once data is collected, it often requires cleaning to remove inaccuracies, duplicates, or irrelevant entries. This step ensures the data’s integrity and reliability. - Exploratory Data Analysis (EDA)
EDA involves examining data sets to summarize their main characteristics. Analysts use statistical tools and visualizations to identify trends, anomalies, and potential areas for deeper analysis. - Data Modeling and Interpretation
Data modeling involves applying algorithms or statistical models to the data to predict outcomes, classify information, or identify patterns. This step transforms raw data into actionable insights. - Visualization and Reporting
Communicating findings effectively is crucial. Data analysts use tools like Tableau, Power BI, and Python libraries to create compelling visualizations that make data understandable for non-technical audiences.
Key Tools and Technologies in Data Analysis
The rise of advanced tools has revolutionized data analysis, making it more accessible and efficient. Some of the most popular tools include:
- Excel: Ideal for basic data manipulation and visualization.
- SQL: Used for querying and managing relational databases.
- Python and R: Programming languages favored for statistical analysis, machine learning, and data visualization.
- Tableau and Power BI: Platforms for creating interactive dashboards and reports.
- Big Data Technologies: Tools like Apache Hadoop and Spark are essential for analyzing massive datasets.
Applications of Data Analysis Across Industries
- Healthcare
Data analysis in healthcare helps predict disease outbreaks, improve patient care, and optimize operational efficiency. For instance, predictive analytics can identify high-risk patients and suggest preventative measures. - Finance
Financial institutions rely on data analysis to detect fraud, assess credit risk, and optimize investment strategies. Real-time analytics helps in monitoring market trends and making swift decisions. - E-Commerce
Online retailers use data analysis to personalize customer experiences, predict buying behaviors, and optimize supply chain management. - Education
In education, data analysis helps track student performance, predict outcomes, and tailor educational content to individual needs. - Sports
Teams leverage data analytics to evaluate player performance, develop strategies, and enhance fan engagement through personalized experiences.
The Importance of Ethical Data Analysis
As data becomes a valuable commodity, ethical considerations in data analysis have gained prominence. Analysts must ensure data privacy, avoid biases in their models, and present findings transparently. Regulatory frameworks like GDPR and CCPA underscore the importance of responsible data handling.
The Future of Data Analysis
The future of data analysis lies in automation and artificial intelligence. Machine learning algorithms and AI-driven tools are enabling analysts to process vast amounts of data in real time. Furthermore, the integration of augmented analytics, which combines AI with human expertise, promises to make data analysis more intuitive and accessible.
Conclusion
Data analysis is more than just numbers and statistics; it is a powerful tool that drives innovation, solves complex problems, and transforms industries. As the world continues to generate more data than ever before, mastering the art and science of data analysis will remain a cornerstone of progress. By leveraging the right tools, methodologies, and ethical practices, data analysts can unlock the full potential of data, paving the way for a smarter and more informed future.