The Importance of Data Analysis in Decision-Making
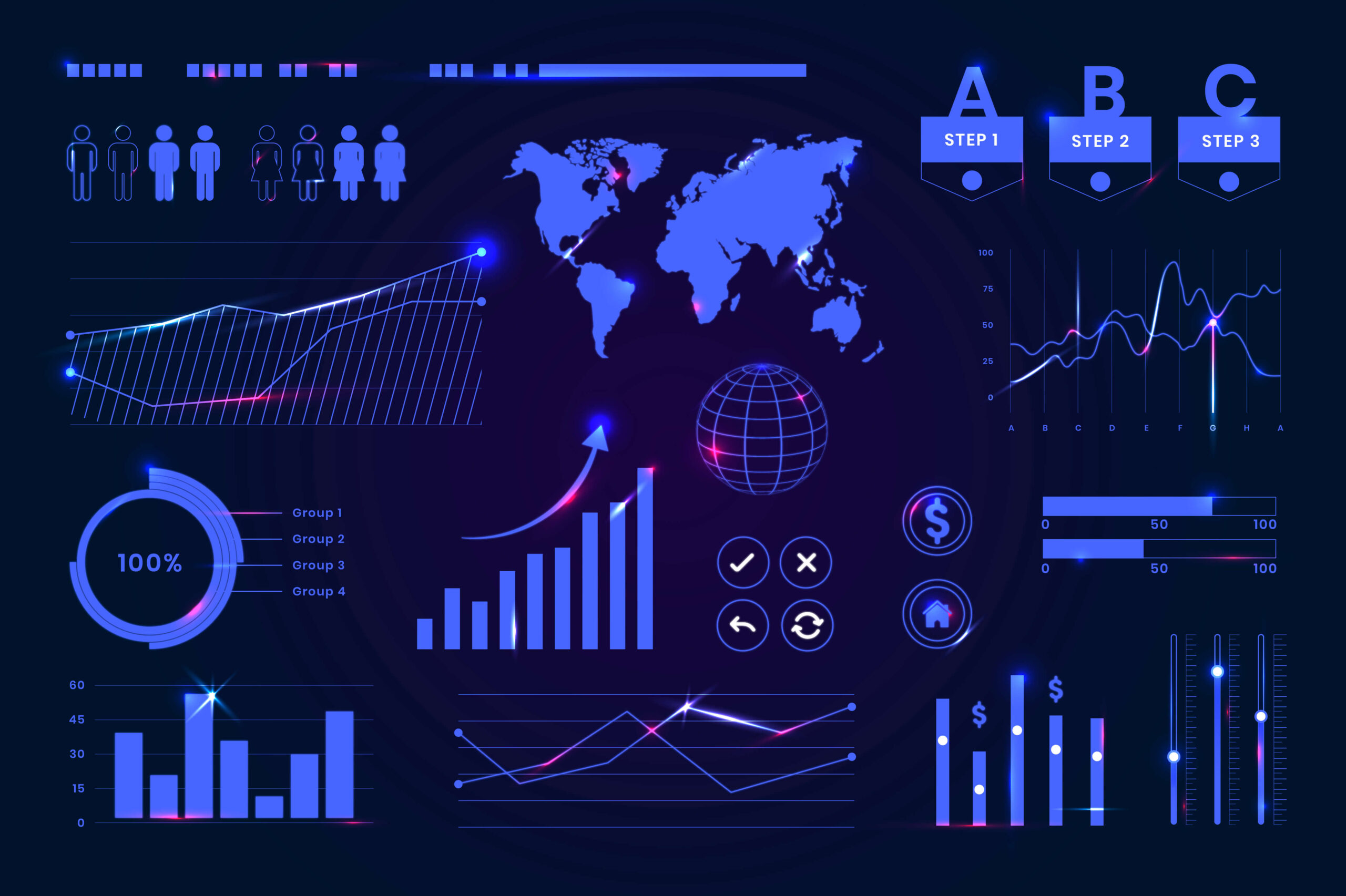
In an era where data is often referred to as the “new oil,” the role of data analysis in decision-making cannot be overstated. Organizations across all industries rely on data analysis to uncover patterns, predict trends, and make informed decisions. This article explores why data analysis is a critical tool for decision-making, the steps involved, and its applications in real-world scenarios.
Why Data Analysis Matters in Decision-Making
- Improved Accuracy
Data analysis eliminates guesswork by providing factual insights, ensuring decisions are based on reliable information. - Risk Reduction
By analyzing historical data, organizations can identify potential risks and devise strategies to mitigate them. - Efficiency
Data analysis streamlines processes by identifying inefficiencies and recommending optimized workflows. - Competitive Advantage
Companies that leverage data analysis gain a competitive edge by predicting market trends and responding proactively. - Customer Insights
Understanding customer behavior through data allows businesses to tailor their offerings, improving customer satisfaction and loyalty.
Steps in Data Analysis for Decision-Making
- Defining the Problem
Clear identification of the problem or decision to be made is the first step. Without a well-defined objective, the analysis can become unfocused. - Data Collection
Gather relevant data from various sources, such as surveys, transaction logs, social media, or internal databases. - Data Cleaning
Raw data often contains errors, duplicates, or missing values. Cleaning the data ensures the analysis is accurate and reliable. - Exploratory Data Analysis (EDA)
Use statistical tools and visualizations to understand the data’s characteristics, identify patterns, and form hypotheses. - Modeling and Analysis
Apply algorithms or statistical models to analyze the data. This step may include regression analysis, classification, clustering, or predictive modeling. - Interpreting Results
Analyze the findings in the context of the problem. Ensure the insights are actionable and aligned with organizational goals. - Decision Implementation
Use the insights to guide decisions, implement strategies, and monitor outcomes to refine future analyses.
Applications of Data Analysis in Decision-Making
- Business Strategy
Companies use data analysis to identify market opportunities, optimize pricing strategies, and plan product launches. - Healthcare
Hospitals analyze patient data to improve treatment protocols, predict disease outbreaks, and optimize resource allocation. - Education
Educational institutions use data to track student performance, predict dropout rates, and enhance teaching methods. - Supply Chain Management
Data analysis helps optimize inventory levels, predict demand, and reduce operational costs. - Sports
Teams leverage data to analyze player performance, develop game strategies, and enhance fan engagement.
Challenges in Data Analysis
While data analysis offers immense benefits, it is not without challenges:
- Data Quality Issues
Poor-quality data can lead to incorrect conclusions and flawed decisions. - Overwhelming Volume
Handling large datasets can be daunting without the right tools and expertise. - Bias and Misinterpretation
Analysts must ensure that biases do not influence the analysis and that findings are interpreted correctly. - Privacy Concerns
Organizations must handle data ethically and comply with regulations to protect sensitive information.
The Role of Technology in Data Analysis
Modern technology has revolutionized data analysis, making it faster and more efficient:
- Artificial Intelligence (AI)
AI-driven tools can process massive datasets, uncover hidden patterns, and provide real-time insights. - Big Data Analytics
Platforms like Apache Hadoop and Spark enable the analysis of large and complex datasets. - Cloud Computing
Cloud-based analytics solutions allow organizations to store, process, and analyze data without significant infrastructure investments. - Visualization Tools
Tools like Tableau, Power BI, and Python libraries create compelling visualizations that make data understandable for stakeholders.
Conclusion
Data analysis has become an indispensable part of decision-making in the modern world. By transforming raw data into actionable insights, organizations can navigate complexities, adapt to changes, and achieve their goals more effectively. However, to harness its full potential, businesses must prioritize data quality, invest in advanced tools, and foster a data-driven culture. As the reliance on data continues to grow, mastering data analysis will remain a cornerstone for success in any field.